A prospective study for predictors of HIV viral load rebound in an HIV hyperendemic rural population of KwaZulu-Natal, South Africa
Emmanuel Abraham Mpolya
Corresponding author: Emmanuel Abraham Mpolya, Department of Global Health and Bio-Medical Sciences, School of Life Sciences and Bioengineering, Nelson Mandela African Institution of Science and Technology, Arusha, Tanzania
Received: 11 May 2023 - Accepted: 23 Jul 2023 - Published: 02 Aug 2023
Domain: Biostatistics,HIV epidemiology
Keywords: HIV, viral load, rebound, suppression, AIDS, recurrent-events, KwaZulu-Natal
©Emmanuel Abraham Mpolya et al. PAMJ-One Health (ISSN: 2707-2800). This is an Open Access article distributed under the terms of the Creative Commons Attribution International 4.0 License (https://creativecommons.org/licenses/by/4.0/), which permits unrestricted use, distribution, and reproduction in any medium, provided the original work is properly cited.
Cite this article: Emmanuel Abraham Mpolya et al. A prospective study for predictors of HIV viral load rebound in an HIV hyperendemic rural population of KwaZulu-Natal, South Africa. PAMJ-One Health. 2023;11:13. [doi: 10.11604/pamj-oh.2023.11.13.40381]
Available online at: https://www.one-health.panafrican-med-journal.com/content/article/11/13/full
Research 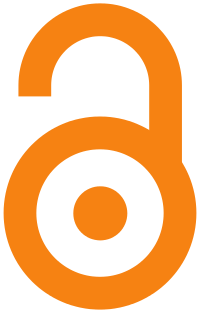
A prospective study for predictors of HIV viral load rebound in an HIV hyperendemic rural population of KwaZulu-Natal, South Africa
A prospective study for predictors of HIV viral load rebound in an HIV hyperendemic rural population of KwaZulu-Natal, South Africa
&Corresponding author
Introduction: globally, up to one third of people living with HIV had unsuppressed viral loads in 2020, making it imperative to understand the various predictors of HIV viral load rebound (HVLR) in specific hyperendemic subpopulations. The objectives of this study were threesome: to determine the period prevalence of HIV viral load rebound in the study population; to describe the association between categorical predictor variables and the geometric mean population viral load (GMVL) and finally to quantify the role of each predictor in determining the hazards of HIV-viral load rebounds in this community.
Methods: data from a prospective cohort study from one of Africa´s largest population-based HIV surveillance in KwaZulu-Natal, South Africa was used to understand predictors of HVLR. Data from HIV-positive individuals who had viral load measurements as well as a suppressed VL followed by at least one viral load measurement between 2011-2018 was analyzed descriptively and inferentially using univariable and multivariable recurrent-events regressions to understand predictors of HVLR. Data management and analyses were done using the R-software and statistical significance was evaluated at the p-value of 0.05.
Results: from a total of 9467 individuals who had at least one viral load measurement, rebound events were observed in 1165 (12.3%) individuals, reflecting falling short of the last 95 of UNAIDS 95-95-95 strategy by 2030. Characteristics such as age, sex, education, marital status, place of residence and antiretroviral (ART) adherence were predictive of hazards of HVLR in both univariable and multivariable analyses. In a multivariable model, hazards of HVLR decreased with increasing age (p < 0.05), increased with being male by 41% (aHR: 1.406; 95% CI: 1.206, 1.639), decreased with having primary education by 22% (aHR: 0.777; 95% CI: 0.612, 0.986), increased with being single by 26% (aHR: 1.259; 95% CI: 1.064, 1.491), decreased by living in rural by 13% (aHR: 0.872; 95% CI: 0.767, 0.992) and decreased with ART adherence by 17% (aHR: 0.834; 95% CI: 0.744, 0.934).
Conclusion: in this setting, the prevalence of HVLR was high, at 12.3% reflecting falling short of the last 95 of UNAIDS 95-95-95 strategy and HVLR was independently associated with being younger, male, having no education, being single, living in rural areas and being ART-adherent. These predictors must be considered in HIV intervention packages to achieve the last 95 of the 95-95-95 HIV-AIDS strategy by 2030 in this setting.
Achievement by 2030 of the UNAIDS targets of diagnosing 95% of all people living with HIV to initiating 95% of all diagnosed people on antiretroviral therapy (ART) and achieving virologic suppression for 95% on ART [1] is partly dependent on how successful is the 'last 95' (of achieving virologic suppression for 95% of all people living with HIV (PLWH) on ART). The efficiency of ART in controlling HIV is well documented [2] but central to its effectiveness is the adherence of patients to ART [3]. Lack of ART adherence reduces the blood concentration of antiretroviral agents leading to rebounds in plasma viral loads, acceleration of HIV drug resistance and high possibility of transmission between an HIV-positive and an HIV-negative individual [4-7] South Africa has the world´s highest number of people living with HIV/AIDS (PLWH) in the world; in 2019, there were 7.7 million PLWH. In response to this situation, the country has the largest antiretroviral treatment (ART) programme with implementation of the Universal Test and Treat (UTT) guidelines since September 2016. The UTT calls for first-line antiretroviral treatment initiation among all known HIV-positive patients, irrespective of their CD4 cell counts [8]. Despite implementation of UTT, there are still situations when the viral load suppression is not achieved because of many factors. For example, individuals initiated on ART under UTT tend to have higher rates of loss to follow up, as the patients start treatment in better health thus failing to perceive direct benefits of treatment [9]. This failure to perceive the benefits of treatment deters patients from consistent engagement in HIV treatment programmes [8]. While there is strong evidence that high ART coverage reduces individuals´ risk of HIV acquisition [2], the loss-to-follow up under UTT is partly responsible for the modest decline in HIV incidence insufficient for the elimination of the HIV/AIDS pandemic in Sub-Saharan Africa despite the widespread rollout and uptake of ART [10].
The indicator of success or failure to achieve the last 95 is the frequency of HIV viral load rebound. A person on ART is said to be in viral load rebound when he or she has persistent, detectable levels of HIV in their blood after a period of undetectable levels. Staying in a virally suppressed state is important as it preserves the immune system, reduces infectivity and prevents the progression or worsening of the AIDS disease [11]. Currently, most of our understanding of the factors affecting HIV viral load rebound are based on predominantly cross-sectional studies [12] or retrospective hospital charts review studies [13,14] of short durations. Our understanding of factors in long-term cohorts is therefore limited. Furthermore, literature review shows that predictors of HVLR are likely to differ depending on study context, data type and analytical approach used. Therefore there is a need to study predictors of HVLR in as many contexts as we can possibly have access to information thereof in order to achieve a comprehensive understanding of drivers of HVLR. The goal of the current study is to understand the prevalence of HVLR and factors affecting it in one of the longest HIV cohorts in the world from an HIV-positive hyperendemic community of KwaZulu-Natal in South Africa [15]. To achieve our goal, the current study followed up a cohort of HIV-positive people from 2011 to 2018 to understand the following three specific objectives: to determine the period prevalence of HIV viral load rebound in the study population throughout the study period; to describe the association between categorical predictor variables and the geometric mean viral load (GMVL) of the study population and finally to quantify the role of each predictor in determining the hazards of HIV-viral load rebounds in this community. The three research questions can be stated as follows: (i) What is the period prevalence of HIV viral load rebound in the study population during the study period? (ii) What is the association between categorical predictor variables and the geometric mean viral load (GMVL) of the study populations? (iii) And finally, what is the role of predictor variables in determining the hazards of HIV viral load rebounds in the study population?
Study design: this was a prospective cohort study design from long-running demographic surveillance systems in South Africa. This demographic surveillance system was started in 2000s with the establishment of the Africa Centre Demographic Information System (ACDIS) [15].
Setting, recruitment and sampling of dried blood spots (DBS): the study area is located near the market town of Mtubatuba in the Umkanyakude district of KwaZulu-Natal located between latitudes of 28° 8' 59" S and longitudes of 32° 12' 0" E. The area is 438 km2 in size and includes a population of between 87,000 to 90,000 people who are members of about 11,000 households [16,18]. The landscape of the study setting is predominantly rural with scattered households. There are several peri-urban settlements and one urban township. Between 2003 and 2006 all women aged 15-49 years and men aged 15-54 years resident in the surveillance area who were eligible for HIV testing [15] were recruited. From 2007 eligibility for the HIV survey was extended to cover all residents aged 15 years or more [2]. From 2011 under the Africa Health Research Institute (AHRI) Population Intervention Program (PIP) Community Viral Load Observation Project [19], all those contacted during the annual surveys were not only tested for HIV but also were consented to provide dried blood spot (DBS) samples. Dried blood spot samples for individuals in the surveillance were collected twice a year and transported to laboratories in Durban where the DBS samples were used to determine viral loads after nucleic acid extraction using the Generic HIV Viral Load kit (Biocentric) [20]. The extraction method has a lower detection limit of 1550 copies/ml [21]. Other details of the survey are provided elsewhere [15,18].
Participants and eligibility criteria: in this study the main goal was to analyze predictors of HIV viral load rebound in an HIV-positive cohort, the eligibility criteria consisted of all persons aged from 15 years onwards who were HIV-positive, had consented to take part in the longitudinal follow-up study and had any viral load measurement at all during the follow-up period. The study population consisted of all residents aged 15 years or more who were HIV-positive between 2011 and 2018 and who had suppressed VL (defined as two consecutive VL tests which were below the suppression cut-off of 1550 copies/ml followed by at least one viral load measurement afterwards.
Ethical approval and consent process: ethical clearance for data collection and use was granted by the biomedical and ethics committee (BREC) of the University of KwaZulu-Natal (Durban, South Africa), with BREC approval number is BE290/16. The consent forms were translated into the Isizulu language and was read to the participants by the field personnel. Any questions about the surveillance were clarified. For participants who could not read and write a helper read out the consent form for them and asked for their consent. They would then consent by making their mark in the consent document. Participants were assured of the privacy and confidentiality of the information they provided.
Data sources, variables, and measurements: the source of data for this study came from the data repository of the Africa Health Research Institute (AHRI) [22]. This repository has data from different surveillances conducted in different parts of South Africa. The author had access to three datasets, namely: AHRI Surveillance Episodes Extended Release 2019 which provided most of the sociodemographic information; the AHRI Core Dataset called Bounded Structures Release 2019 which provided spatially related data and the Community Viral Load Dataset which contained results from the dried blood spots (DBS) analyses [22]. All these datasets had common identifiers, which could enable the linking of different information pertaining the participant. Viral load status data was linked with other variables such as age, sex, household role, education, marital status, distance to the nearest clinic and place of residence. Age was recorded in complete years during the surveillance episode in a particular year and was later categorized into 10-years intervals as (15-24), (25-34), (35-44), (45-54) and 55 years and above. The basis of this categorization was on reflecting the major stages of development and aging [23]. Sex of an individual had three categories: female, male and unknown, there were no individuals with unknown gender information and so it was dichotomized into male and female categories. Household role was modified from the variable called relationship to the head of the household in which various relationship states to the head of the household were recorded, it was dichotomized into either head or non-head of the household. Education was recorded as the education status during a particular surveillance episode and was recorded either as none or per educational grades in South Africa. During analysis, education was categorized as none, primary, secondary and tertiary. The recorded marital status was the one during the given surveillance episode and was categorized as informal union, married, separated and single. Distance to nearest clinic was spatially derived after the participant had named the nearest clinic during the survey and place of residence was derived from a variable which identified whether a participant was coming from the rural, peri-urban or urban settings.
The outcome variable was time to HIV viral load rebound (HVLR). Viral load rebound was defined as having a detectable viral load (>1550 copies/ml) following a state of suppression (≤1550 copies/ml). Each participant who had at least one documented viral load measures between 2011 and 2018 were selected, and viral suppression was affirmative when an individual had two consecutive viral load tests which were below the suppression cut-off. These suppressed individuals were followed-up until occurrence of loss-to-follow up or a viral load rebound. In this context, one participant could have multiple viral load rebounds during the follow-up period. During the process of linking the data, there were instances of missing data which were excluded in this analysis since the purpose was to analyse individuals who had completed follow-ups for the 8 years to understand the predictors of HVLR. This action would not affect the external validity, which is generalization to people with HIV/AIDS.
Study size and power analysis: the sample size was obtained from the criteria set for data analysis in this study. The criteria were to include individuals who had any viral load measurement at all during the follow-up period. In the next section, we provide the details to show that this sample size has enough power to reject false null hypotheses. Towards this end, the minimum sample size which has a power of 80% at a Type 1 error of 5% and 95% Confidence Interval within 5% of true population parameters is calculated. Then the minimum sample obtained is compared to the sample size in this study. If the latter is higher than the former, then the latter is deemed to have enough power. Power analysis was done using the cpower function in the R package Hmisc [24]. The cpower function takes the following arguments: the time period during which outcome events were estimated, which was 8 years, the probability of HIV viral load rebound among those who were HIV-viral load suppressed was taken to be non-zero but negligibly small. The lifetime probability of HIV viral load rebound among those who had rebound events was taken as 12.3% (calculated from this study). The ratio of population having viral load rebound to that not having it, which is 1165:4949 is almost 1:4. With these parameters, the sample size that has the power of 80% to reject false null hypothesis and estimate population parameters within 5% of the true parameters and with a Type 1 error of 5% and 95% Confidence Interval of 97% was 650. For multivariable Cox regressions, it is advisable to perform a simulation of the power of the obtained sample size. Simulations for the power of the sample size of 650 were repeated 1000 times using the spower function from the Hmisc package [24] which gave the power of almost 100%. Therefore, since the required samples size is 650 and the sample size of this study is 9467, there is sufficient power to find differences that exist in this study population.
Data management and statistical analysis: data management involved merging data from the three datasets namely: AHRI Surveillance Episodes Extended Release 2019 which provided most of the sociodemographic information; the AHRI Core Dataset called Bounded Structures Release 2019 which provided spatially related data and the Community Viral Load Dataset which contained results from the dried blood spots (DBS) analyses[22]. Descriptive analyses for demographic and other variables against HIV viral load status as well as rebound status were calculated. Cross-tabulation between various categorical predictor variables was done and all variables which had statistically significant chi-squared statistics (p-value < 0.05) were included in the regression models. Then survival analytical unadjusted (univariable) and adjusted (multivariable) regression analyses to examine the crude and independent effects of each predictor variable in determining the hazards of HVLR, respectively, were done. In this study, ‘survival´ was defined as the time in a state of HIV viral suppression, while the ‘event´ was the HIV Viral Load Rebound (HVLR) [25]. Since data in this study tracks one person over time, then the survival analytical framework used was the recurrent-events framework using the Prentice, Williams and Peterson (total time) (PWP-TT) model which allows for variations of effects of covariates from event to event and takes into consideration the total follow-up time [26]. The PWP-TT model took into consideration the clustering due to one individual being followed up over time, and stratification was handled in terms of rebound events. All predictor variables were analysed in both the un-adjusted and adjusted survival analytical regression frameworks. The p-value of 0.05 was used as a cut-off value for statistical significance. All analyses were done using the R statistical software [27].
Data analysis was targeted to answer the three research questions: what is the period prevalence of HIV viral load rebound in the study population? What is the association between categorical predictor variables and the geometric mean viral load (GMVL) of the study populations? And finally, what is the role of predictor variables in determining the hazards of HIV viral load rebounds in the study population? In the subsequent sections results to these questions are presented.
Period prevalence of HIV viral load rebound in the study population: the denominator for the period prevalence of HIV viral load rebound in this study population is the total study population who had any viral load measurement at all, which was 9467 individuals. To obtain its numerator, which were individuals with one, two, or three HIV viral load rebounds, we performed the following procedure. Firstly, individuals who had any viral load measurements at all were selected, followed by exclusion of all individuals who had only one viral load measurements throughout the study period. Thereafter, from among individuals who had more than one viral load measurements, exclusion of all individuals who did not have an HIV viral load rebound at all was done. This action ensured that a sample of individuals with either one, two, or three viral load rebounds was obtained. This is detailed in Figure 1. This left 1165 individuals who had either one, two or three viral load rebounds making the period prevalence of HVLR to be 12.3%. Indeed, 1165 is the number of outcome events, which in this case is the HIV viral load rebounds measured between 2011 until 2018 in this study population.
Demographic characteristics of the study population at baseline: the study population was relatively young, with a median age of 38 years (IQR: 30 - 49). The summary of other demographic characteristics is shown in Table 1. About 70% of the study population was aged between 15 years and 44 years, inclusive. The study population was majority young people. In this study, members of the female sex are predominant, being about 82% of the population. Majority of the participants had a secondary education (about 73%). About 82% of the participants were either in an informal union or single, and only about 9% were married. About 9.5% were separated. Household power dynamics are important in African settings as they reflect who is in control of the household resources. Majority of participants were non-heads of households (32%). In terms of place of residence, the majority of the participants came from the rural areas (67%) as the study location is predominantly rural with only pockets of urban and peri-urban locations. Adherence to antiretroviral therapy (ART) is an important determinant of HVLR, and in this population, about one third of participants were not on ART. About 50% of the study population was living within 3 km from the nearest clinic.
Relationship between categorical predictor variables and the geometric mean viral load (GMVL): the distribution of viral load readings can be extremely skewed (with quite large fluctuations) and for repeated measures of viral loads the readings are not independent making the application of the geometric mean ideal in these circumstances [28]. Table 2 shows the cross-tabulation between categorical predictor variables and the geometric mean population-based viral loads. The 95% Confidence Intervals of the geometric mean viral load (GMVL) is presented as well as the test of association between the different levels of the categorical variables and the GMVL. The highest geometric mean viral load among age categories is within the 15-24 age range, even though they only represented 13.9% of the total population (Table 1). There is a significant association between GMVL and the ages. With respect to the sexes, males, who only represented 18% of the total population, had higher geometric mean viral load than the females and GMVL seems to be associated with sexes. Moreover, the highest GMVL within the education variable was among those with secondary education, who are also highly represented as well. There seems to be an association between the level of education and the GMVL. Marital status seems to have an influence on the level of GMVL, and GMVL is highest among those in informal union, followed by those who are single. Position in the household seems to have an association to the GMVL, and the GMVL is highest among non-heads of households. Despite the fact that the majority of participants came from the rural areas, the highest GMVL was among those living in urban areas, even though they were just 7.5% of the total population. Finally, with respect to adherence to ART, those who were not on ART had higher GMVL than those who were on ART (Table 2).
Role of predictor variables in determining the hazards of HIV-viral load rebounds
Univariable analysis results: Table 3 shows results from the univariable PWP-TT model. This model evaluated the effect of each predictor on the hazards of HVLR and summarized such hazards using the un-adjusted (or crude) hazard ratio. The PWP-TT model controlled for the clustering effects due to individuals and controlled the repeated HIV-viral load rebounds by the stratification of such HIV viral load rebounds. It is observed that a unit increase in age lowers the hazards of HVLR by 1% (HR: 0.99; 95% CI: 0.986, 0.994), indicating that older age individuals stay longer in a state of HIV viral suppression than younger age individuals. Upon categorization of age groups, a similar result was echoed in which we observed that, compared to the 15-24 years age group, older age groups had decreased hazards of HVLR. In this univariable framework, males have higher hazards of HVLR than females by 32% (HR: 1.32; 95% CI: 1.137, 1.532). With respect to education level, only the primary education seems to be protective of HVLR compared to no education (HR:0.715; 95% CI: 0.566, 0.904). With the marital status, compared to people in informal union, only people who are separated (HR: 0.791; 95% CI: 0.670, 0.934) had significant protection from HVLR, by about 21%. ART adherence influenced the hazards of HVLR, with people on ART being protected with a 12% reduction in the hazards of rebound (HR: 0.879; 95% CI: 0.787, 0.981). Finally, position within the household, place of residence and distance to nearest clinic did not have any influence on the hazards to HVLR (p > 0.05).
Multivariable analysis results: while univariable analysis considered the effect on the outcome by only one independent variable at a time, the multivariable analysis considers the effects of more than one independent variable on the outcome of interest. The effects of independent variables on the outcome variable are thus those effects when all other co-variates are controlled. Multivariable analyses help us to understand potential confounding as well as interactions between variables. Table 4 shows results from the multivariable PWP-TT model using all predictors in the univariable models. Results from this model show that age is still a significant predictor of HVLR, a unit increase of which decreasing the hazards of HVLR by 12% (HR: 0.982; CI: 0.967, 0.998). When age is categorized into groups, there is no significant effect on the hazards of HVLR because categorization is known to decrease the power compared to the continuous version of an independent variable. The following demographic and spatial variables such as sex, education, marital status, place of residence and ART adherence have independent effects on the hazards of HVRL as follows. Compared to females, the hazards of HVLR among males are almost 41% more (HR: 1.406; 95% CI: 1.206-1.639). With respect to education, when compared to participants with no education, people with primary education have a reduced hazard of HVLR by almost 22% (HR: 0.777; 95% CI: 0.612, 0.986). With marital status, compared to those in informal union, participants who were single were almost 26% more likely to experience HVLR (HR: 1.259; 95% CI: 1.064, 1.491). People living in rural areas have about 13% reduction in the hazards of HVLR compared to those in peri-urban (HR: 0.872; 95% CI: 0.767, 0.992). Importantly, participants who were ART adherent were protected from HVLR by up to 17% compared to those who were not ART adherent (HR: 0.834; 95% CI: 0.744, 0.934). Finally, position in the household and distance to the nearest clinic did not have any statistically significant effects on the hazards of HVLR (p-value > 0.05).
This study was aimed at achieving three objectives: to determine the period prevalence of HIV viral load rebound in the study population during the study period; to describe the association between categorical predictor variables and the geometric mean viral load (GMVL) of the study population and finally to quantify the role of each predictor in determining the hazards of HIV-viral load rebounds in this community. With respect to the first objective, the period prevalence of HVLR found in thi study is 12.3% which is higher than the recently reported rate of 7.5% in the USA [29]. In another South African study where electronic medical records of people living with HIV (PLWH) from 2004 to 2016 were studied, the prevalence of unsuppression was reported as 15% [14]. A Ugandan study to understand factors for HIV viral load suppression in a district hospital reported a prevalence of unsuppression of 14.3% [13] while a similar study in Ghana reported the prevalence of 19% [12]. In another retrospective cohort study in Providence, Rhode Island, USA, it was reported that only 2% - 5% of the participants experienced viral load rebounds [30]. These difference underlines the importance of understanding specific subpopulation context to understand the predictors of HVLR. The period prevalence of HVLR of 12.3% reported in this study is still high and reflects the falling short of achieving the last 95% of the UNAIDS 95-95-95 strategy. This high prevalence of HVLR could be attributed to various reasons, socio-economic development and quality of healthcare could be among of the reasons. This study adds to the information package about the potential predictors of this situation.
The affected study population was relatively young with a median age of 38 years reflecting the impact of HIV/AIDS in decimating the most productive age brackets, something which has been corroborated by a recent South African study [14]. Moreover, another recent study to update on the age distribution of new HIV infections and how this distribution changed over reporting the age at infection among men and women to be between 24.8 years and 34.6 years [31], again heavily affecting the young people. Objectives two and three were addressed in cross-tabulations and survival analytical regression analyses. Starting with cross-tabulations, all the predictor variables were significantly associated with the differences in geometric mean viral loads (GMVL) (p < 0.0001) reflecting the importance of doing a comprehensive assessment of different predictors when planning for interventions in HIV/AIDS care and treatment
With regards to survival analytic regressions, demographic predictors including age, sex, education and marital status were significantly associated with hazards to HVLR in both univariable and multivariable regression analyses. Therefore, in general, younger individuals are at a higher hazard of HVLR which was similarly reported by another recent South African study by Davey et al. [14]. However, other commentators in South Africa have contrasting results where an increase in age was associated with an increase in the risk of HVLR (mediated by poor adherence to ART and social behaviors such as alcoholism, which generally increase with age) [32]. Among several other factors to cause HVLR such as drug-drug interactions, treatment failure, drug resistance and unobserved factors; it is also conjectured that HVLR is possibly associated with poor ART adherence [33]. This study also finds a significant protective effect of ART adherence to HVLR similar to the study by Morojele et al. [32] and Bulage et al. [33]. Results from this study are also similar to findings from a comparative study between African and Asian cohorts which associates young age to sub-optimal degrees of ART adherence and therefore higher hazards of HVLR [34]. Results from this study are also similar to another study from Ethiopia which reported that an increase in age was associated with a reduction in hazards of CD4 count change [35]. Besides, a Ugandan study, despite being a cross-sectional design, came with a similar conclusion that an increase in age was associated with a reduction in hazards of viral load rebound [33]. A recent modelling study has also cited younger age as being significantly associated with HVLR [36]. Younger HIV-positive patients are most likely to be socio-economically unstable [37], occupied with activities to earn a living or create a better future and therefore more likely to fall short in adherence to ART, increasing their hazards of HVLR.
This study reports that being of male sex carries a higher hazard of HVLR in agreement with previous studies. For example, three studies have reported that in the African setting being of male sex is a determinant of sub-optimal ART adherence [14,34,35] thus increasing the hazards to HVLR. Furthermore, a study by Seyoum et al. also reported similar conclusion of higher hazards of VLR among males. In contrast, a South African study reported no effect of gender in affecting the transition between a state of HVLR and HIV viral suppression [38]. In contrast to our findings, a nationwide Asian study associated poor adherence to ART with being a female [37]. These diverse findings about the effect of sex on the hazards of HVLR are a pointer to the importance of context in understanding the predictors of HVLR. We find an independent effect of education, in which having a primary education is protective from hazards of HVLR. Similar findings of a positive effect of education to survival in virally-suppressed state have been reported elsewhere [35]. Lower education level as predictors of hazards of HVLR has also been reported by a recent modelling study [36]. Therefore, more innovative health education methods, such as those with tailored local languages, would be useful to empower those who are completely uneducated.
Another demographic for which we find independent effect in determining hazards of HVLR is marital status, in which being single is hazardous of HVLR. This is in agreement with other studies which have reported being young and unmarried as sources of hazards of viral load rebound [32,33,35]. A recent modelling study has reported that having a stable partner is a predictor of lower hazards of HVLR [36], supporting our current findings, since the opposite of having a stable partner is being single and unmarried. Single people may lack the communication benefits and the social support advantages that sometimes come when you have a partner who understand your HIV-status which have been reported to improve ART adherence [39,40].
This study found that there is a protective effect against HVLR when one lives in a rural area compared to the peri-urban areas. This is in contrast to a study in the USA which investigated the rural-urban differences in HIV viral loads and found non-significant rural-urban differences in viral load counts at 1 year but then observed more people in the rural were later diagnosed to have or progressed to AIDS [41]. The urban and rural context of the US are surely different from those of the current study, again emphasizing the importance of studying context-specific determinants of hazards. This study did not find any influence on hazards of HVLR due to position in the household or distance to the nearest clinic. With respect to position in the household, a recent study studies patterns of family support on the role in HIV viral load suppression and found that good family support was associated with less risk of HIV viral load rebound [42]. The current study only investigated whether being a head of household or non-head affected the hazards of HVLR, but did not go as methodologically deep as the study by Nakandi et al. mentioned previously. With respect to distance to the nearest clinic, a recent study investigated the roles of travel distance to HIV care clinic on the transmission risk of HIV sero-discordant couples in Henan Province, China and found that travel distance was associated with HIV transmission only after stratification. They found that travelling medium distances to villages increased transmission risks, while travelling longer distances to a township or county lowered the transmission risk [43]. While the current study was not focused on HIV transmission, the previously Chinese study does imply that distance to the clinic might have some bearing on affecting some aspects of HIV/AIDS outcomes.
Limitations of this study include the missingness in the data. This being a follow-up and open cohort study, several individuals were not all followed up throughout the study period, leading to missing data. This forced us to include only variables with no missingness or less than 1% of missingness. Other potentially important variables such as economic, water and sanitation were thus not included. The strength of this study lies in its design of being a prospective cohort study and using data from one of the world´s largest on-going population-based cohorts in rural KwaZulu-Natal, South Africa. Previously, data from this cohort have provided some of the most important insights in the HIV-AIDS pandemic to date [2,44]. In this study, we not only followed up over time, but also, we followed-up each individual as they experienced viral suppressions and rebounds and employed recurrent events analytical methods to capture more realistic aspects of the events. From this point of view, our findings should be an important addition to the knowledge about HIV-AIDS control and interventions and should be generalizable to similar settings in sub-Saharan Africa.
Predictors of hazards to HVLR in a recurrent-events framework are mainly demographic, namely: being of young age, of male sex, having no education and being single. HIV-AIDS intervention packages in this hyperendemic rural population of KwaZulu-Natal, South Africa, must take these predictors into consideration to achieve the last 95 of the 95-95-95 HIV-AIDS strategy by 2030.
What is known about this topic
- By 2030, the UNAIDS targets diagnosing 95% of all people living with HIV, then initiating 95% of all diagnosed people on antiretroviral therapy (ART) and achieving virologic suppression for 95% on ART;
- Up to one third of people living with HIV had unsuppressed viral loads in 2020;
- Most research has focused on small populations using cross-sectional study designs or retrospective cohort studies using electronic medical records.
What this study adds
- Updates predictors of HIV viral load rebound using data from one of the longest-running HIV population surveillance in the world;
- Updates the period prevalence of HVLR in a South African HIV hyperendemic population to be 12.3% which reflects falling short of achieving the last 95 of the UNAIDS 95-95-95 by 2030 ;
- Finds a significant association between geometric mean viral loads (GMVL) to socio-demographic and ART-adherence predictors; using survival analytical approaches, quantifies the independent effects of age, sex, education, marital status, place of residence and ART adherence as determinants of hazards to HVLR.
The author declares no competing interests.
Emmanuel Abraham Mpolya conceived the study, managed, analyzed data and wrote the manuscript. The author have read and agreed to the final manuscript.
Special gratitude is due to the following persons for their contributions to this work at various times when I was a post-doctoral fellow at the Africa Health Research Institute (AHRI) in Durban, South Africa: Dr. Hae-Young Kim; Dr. Maxime Inghels; Dr. Polycarp Mogeni; Dr. Okango Elphas; Dr. Andrew Tomita; Prof. Till Baernighausen; Prof. Tulio de Oliveira and Prof. Frank Tanser. The Grant Manager, Ms. Thulile Mathenjwa, is also hereby duly acknowledged. Also, gratitude to the National Institute of Health (NIH) grants (R01HD084233 and R01AI124389) from the National Institute of Child Health and Human Development (USA) and the Wellcome Trust, (UK) for funding my Post-doctoralship at the Africa Health Research Institute (AHRI) in Durban, South Africa.
Table 1: demographic and ART adherence characteristics of the study population at baseline
Table 2: relationship between categorical predictor variables and the geometric mean viral loads (GMVL) with tests of independence
Table 3: univariable Recurrent-events Prentice, Williams and Peterson (total time) Cox regression models (PWP-TT), outcome variable is time to HVLR
Table 4: multivariable Recurrent-events Prentice, Williams and Peterson (total time) regression models (PWP-TT), outcome variable is time to HVLR
Figure 1: flow chart for obtaining the denominator and numerator for period prevalence of HIV viral load rebound (HVLR)
- UNAIDS. 90-90-90 - An ambitious treatment target to help end the AIDS epidemic. Accessed October 26, 2020.
- Tanser F, Bärnighausen T, Grapsa E, Zaidi J and Newell ML. High coverage of ART associated with decline in risk of HIV acquisition in rural KwaZulu-Natal, South Africa. Sciences. 2013 Feb 22;339(6122):966-71. PubMed | Google Scholar
- Silva JAG, Dourado I, de Brito AM and da Silva CAL. Factors associated with non-adherence to antiretroviral therapy in adults with AIDS in the first six months of treatment in Salvador, Bahia State, Brazil. Cadernos de Saúde Pública. 2015;31(6):1188-1198. PubMed | Google Scholar
- Chaiyachati KH, Ogbuoji O, Price M, Suthar AB, Negussie EK, Bärnighausen T. Interventions to improve adherence to antiretroviral therapy: A rapid systematic review. AIDS. 2014 Mar;28 Suppl 2:S187-204. PubMed | Google Scholar
- Chesney MA. Factors Affecting Adherence to Antiretroviral Therapy. Clinical Infectious Diseases. 2000;30(2)S171-S176. PubMed | Google Scholar
- Quinn TC, Wawer MJ, Sewankambo N, Serwadda D, Li C, Wabwire-Mangen F et al. Viral Load and Heterosexual Transmission of Human Immunodeficiency Virus Type 1. N Engl J Med. 2000 Mar 30;342(13):921-9. PubMed | Google Scholar
- Cohen MS et al. Prevention of HIV-1 Infection with Early Antiretroviral Therapy. New England Journal of Medicine. 2011;365(6):493-505. PubMed | Google Scholar
- Hirasen K, Fox MP, Hendrickson CJ, Sineke T, Onoya D. HIV treatment outcomes among patients initiated on antiretroviral therapy pre and post-universal test and treat guidelines in South Africa. Ther Clin Risk Manag. 2020 Mar 4;16:169-180. PubMed | Google Scholar
- Justman JE, Mugurungi O and El-Sadr WM. HIV Population Surveys - Bringing Precision to the Global Response. N Engl J Med. 2018 May 17;378(20):1859-1861. PubMed | Google Scholar
- Mahy M, Marsh K, Sabin K, Wanyeki I, Daher J, Ghys PD. HIV estimates through 2018: Data for decision-making. AIDS. 2019 Dec 15;33 Suppl 3(Suppl 3):S203-S211. PubMed | Google Scholar
- Phillips AN, Staszewski S, Weber R, Kirk O, Francioli P, Miller V et al. HIV Viral Load Response to Antiretroviral Therapy According to the Baseline CD4 Cell Count and Viral Load. JAMA. 2001;286(20):2560-2567. PubMed | Google Scholar
- Owusu LB, Ababio C, Boahene S, Zakaria AFS, Emikpe AO, Dwumfour CK et al. The predictors of unsuppressed viremia among PLHIV: a cross-sectional study in Ghana. BMC Public Health. 2023;23(1):1113. PubMed | Google Scholar
- Wakooko P, Gavamukulya Y, Wandabwa JN. Viral load Suppression and Associated Factors among HIV Patients on Antiretroviral Treatment in Bulambuli District, Eastern Uganda: A Retrospective Cohort Study. Infect Dis (Auckl). 2020 Nov 5;13:1178633720970632. PubMed | Google Scholar
- Joseph Davey D, Abrahams Z, Feinberg M, Prins M, Serrao C, Medeossi B et al. Factors associated with recent unsuppressed viral load in HIV-1-infected patients in care on first-line antiretroviral therapy in South Africa. International journal of STD & AIDS. 2018;29(6):603-610. PubMed | Google Scholar
- Tanser F, Hosegood V, Bärnighausen T, Herbst K, Nyirenda M, Muhwava W et al. Cohort Profile: Africa Centre Demographic Information System (ACDIS) and population-based HIV survey. International Journal of Epidemiology. 2008;37(5):956-962. PubMed | Google Scholar
- Tomita A, Vandormael A, Bärnighausen T, Phillips A, Pillay D, De Oliveira T et al. Sociobehavioral and community predictors of unsuppressed HIV viral load. AIDS. 2019 Mar 1;33(3):559-569. PubMed | Google Scholar
- Tanser F, Vandormael A, Cuadros D, Phillips AN, De Oliveira T, Tomita A et al. Effect of population viral load on prospective HIV incidence in a hyperendemic rural African community. Sci Transl Med. 2017 Dec 13;9(420):eaam8012. PubMed | Google Scholar
- Vandormael A, Cuadros D, Kim HY, Bärnighausen T, Tanser F. The state of the HIV epidemic in rural KwaZulu-Natal, South Africa: a novel application of disease metrics to assess trajectories and highlight areas for intervention. Int J Epidemiology. 2020;49(2):666-675. PubMed | Google Scholar
- Yapa HM, De Neve JW, Chetty T, Herbst C, Post FA, Jiamsakul A et al. The impact of continuous quality improvement on coverage of antenatal HIV care tests in rural South Africa: Results of a stepped-wedge cluster-randomised controlled implementation trial. PLoS Med. 2020 Oct 7;17(10):e1003150. PubMed | Google Scholar
- Ngo-Malabo ET, Ngoupo TPA, Zekeng M, Ngono V, Ngono L, Sadeuh-Mba SA et al. A cheap and open HIV viral load technique applicable in routine analysis in a resource limited setting with a wide HIV genetic diversity. Virology journal. 2017;14(1):224. PubMed | Google Scholar
- Viljoen J, Gampini S, Danaviah S, Valéa D, Pillay S, Kania D et al. Dried blood spot HIV-1 RNA quantification using open real-time systems in South Africa and Burkina Faso. Journal of Acquired Immune Deficiency Syndromes. 2010;55(3):290-298. PubMed | Google Scholar
- Africa Health Research Institute (AHRI). AHRI Data Repository; data Catalog. Accessed October 26, 2020.
- Geifman N, Cohen R and Rubin E. Redefining meaningful age groups in the context of disease. Age (Dordrecht, Netherlands). 2013;35(6):2357-2366. PubMed | Google Scholar
- Harrel JF. Hmisc: Harrel Miscellaneous. . 2023. Accessed October 26, 2020.
- Vandormael A, Bärnighausen T, Herbeck J, Tomita A, Phillips A, Pillay D, et al. Longitudinal Trends in the Prevalence of Detectable HIV Viremia: Population-Based Evidence from Rural KwaZulu-Natal, South Africa. Clin Infect Dis. 2018 Apr 3;66(8):1254-1260. PubMed | Google Scholar
- Amorim LDAF, Cai J. Modelling recurrent events: A tutorial for analysis in epidemiology. Int J Epidemiol. 2015 Feb;44(1):324-33. PubMed | Google Scholar
- R Core Team. R: A language and environment for statistical computing. R Foundation for Statistical Computing Vienna, Austria. 2016. Google Scholar
- Rose CE, Gardner L, Craw J, Girde S, Wawrzyniak AJ, Drainoni ML et al. A Comparison of Methods for Analyzing Viral Load Data in Studies of HIV Patients. PLoS One. 2015 Jun 19;10(6):e0130090 PubMed | Google Scholar
- Craw JA, Beer L, Tie Y, Jaenicke T, Shouse RL and Prejean J. Viral Rebound Among Persons With Diagnosed HIV Who Achieved Viral Suppression, United States. J Acquir Immune Defic Syndr. 2020 Jun 1;84(2):133-140. PubMed | Google Scholar
- Min S, Gillani FS, Aung S, Garland JM, Beckwith CG. Evaluating HIV Viral Rebound Among Persons on Suppressive Antiretroviral Treatment in the Era of “Undetectable Equals Untransmittable (U = U)”. Open Forum Infect Dis. 2020 Nov 16;7(12):ofaa529. PubMed | Google Scholar
- Risher KA, Cori A, Reniers G, Marston M, Calvert C, Crampin A et al. Age patterns of HIV incidence in eastern and southern Africa: a modelling analysis of observational population-based cohort studies. Lancet HIV. 2021 Jul;8(7):e429-e439. PubMed | Google Scholar
- Morojele NK, Kekwaletswe CT, Nkosi S. Associations between alcohol use, other psychosocial factors, structural factors and antiretroviral therapy (ART) adherence among South African ART recipients. AIDS Behav. 2014 Mar;18(3):519-24. PubMed | Google Scholar
- Bulage L, Ssewanyana I, Nankabirwa V, Nsubuga F, Kihembo C, Pande G et al. Factors Associated with Virological Non-suppression among HIV-Positive Patients on Antiretroviral Therapy in Uganda, August 2014-July 2015. BMC Infect Dis. 2017 May 3;17(1):326. PubMed | Google Scholar
- Bijker R, Jiamsakul A, Kityo C, Kiertiburanakul S, Siwale M, Phanuphak P et al. Adherence to antiretroviral therapy for HIV in sub-Saharan Africa and Asia: A comparative analysis of two regional cohorts. J Int AIDS Soc. 2017 Mar 3;20(1):21218. PubMed | Google Scholar
- Seyoum A, Ndlovu P and Temesgen Z. Joint longitudinal data analysis in detecting determinants of CD4 cell count change and adherence to highly active antiretroviral therapy at Felege Hiwot Teaching and Specialized Hospital, North-west Ethiopia (Amhara Region). AIDS Res Ther. 2017 Mar 16;14(1):14. PubMed | Google Scholar
- Dessie ZG, Zewotir T, Mwambi H, North D. Modeling Viral Suppression, Viral Rebound and State-Specific Duration of HIV Patients with CD4 Count Adjustment: Parametric Multistate Frailty Model Approach. Infect Dis Ther. 2020 Jun;9(2):367-388. PubMed | Google Scholar
- Kim J, Lee E, Park BJ, Bang JH, Lee JY. Adherence to antiretroviral therapy and factors affecting low medication adherence among incident HIV-infected individuals during 2009-2016: A nationwide study. Sci Rep. 2018 Feb 16;8(1):3133. PubMed | Google Scholar
- Shoko C, Chikobvu D. Determinants of viral load rebound on HIV/AIDS patients receiving antiretroviral therapy: Results from South Africa. Theor Biol Med Model. 2018 Jul 16;15(1):10. PubMed | Google Scholar
- Jin H, Biello K, Garofalo R, Lurie M, Sullivan PS, Stephenson R et al. Better Communication Regarding Sexual Agreements Within Serodiscordant Male Couples Leads to Improved Antiretroviral Therapy Adherence Among the HIV-Positive Partner. Archives of Sexual Behavior. 2021;50(4):1771-1779. PubMed | Google Scholar
- Darbes LA, Chakravarty D, Beougher SC, Neilands TB, Hoff CC. Partner-provided social support influences choice of risk reduction strategies in gay male couples. AIDS and Behavior. 2012;16(1):159-167. PubMed | Google Scholar
- Weissman S, Duffus WA, Iyer M, Chakraborty H, Samantapudi AV, Albrecht H. Rural-urban differences in HIV viral loads and progression to AIDS among new HIV cases. Southern medical journal. 2015;108(3):180-188. PubMed | Google Scholar
- Nakandi RM, Kiconco P, Musiimenta A, Bwengye JJ, Nalugya S, Kyomugisa R et al. Understanding patterns of family support and its role on viral load suppression among youth living with HIV aged 15 to 24 years in southwestern Uganda. Health Sci Rep. 2022 Jan 19;5(1):e467. PubMed | Google Scholar
- Smith MK, Miller WC, Liu H, Ning C, He W, Cohen MS et al. Effects of patient load and travel distance on HIV transmission in rural China: Implications for treatment as prevention. PLoS One. 2017 May 31;12(5):e0177976. PubMed | Google Scholar
- Akullian A, Vandormael A, Miller JC, Bershteyn A, Wenger E, Cuadros D et al. Large age shifts in HIV-1 incidence patterns in KwaZulu-Natal, South Africa. Proc Natl Acad Sci U S A. 2021 Jul 13;118(28):e2013164118. PubMed | Google Scholar