External validation and improvement of the CABI prognosis score in Guinean patients treated for tuberculosis in Conakry
Gerard Christian Kuotu, Alhassane Diallo, Boubacar Djelo Diallo, Demba Toure, Lansana Mady Camara
Corresponding author: Gerard Christian Kuotu, Faculty of Health Sciences and Techniques, Gamal Abdel Nasser University in Conakry, Pneumo-Phthisiology Department, Conakry University Hospital, Ignace Deen National Hospital in Conakry, Guinea
Received: 29 Mar 2021 - Accepted: 06 Jan 2022 - Published: 14 Jan 2022
Domain: Infectious disease
Keywords: CABI score, TABI score, tuberculosis, Guinea
©Gerard Christian Kuotu et al. PAMJ-One Health (ISSN: 2707-2800). This is an Open Access article distributed under the terms of the Creative Commons Attribution International 4.0 License (https://creativecommons.org/licenses/by/4.0/), which permits unrestricted use, distribution, and reproduction in any medium, provided the original work is properly cited.
Cite this article: Gerard Christian Kuotu et al. External validation and improvement of the CABI prognosis score in Guinean patients treated for tuberculosis in Conakry. PAMJ-One Health. 2022;7:6. [doi: 10.11604/pamj-oh.2022.7.6.29085]
Available online at: https://www.one-health.panafrican-med-journal.com/content/article/7/6/full
Research 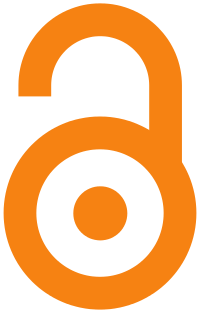
External validation and improvement of the CABI prognosis score in Guinean patients treated for tuberculosis in Conakry
External validation and improvement of the CABI prognosis score in Guinean patients treated for tuberculosis in Conakry
Gerard Christian Kuotu1,&, Alhassane Diallo2,
Boubacar Djelo Diallo1, Demba Toure1, Lansana Mady Camara1
&Corresponding author
Introduction: tuberculosis is an infectious communicable disease caused by mycobacterium tuberculosis, it is the second leading cause of death following Human immunodeficiency virus (HIV).
Methods: to carry out this work, we proposed to validate externally the prognostic score (CABI) developed in Cameroon in Guinean patients treated for tuberculosis then to improve the CABI score (substitution of one or more factors) only if the predictive capacities of the score are not satisfactory (index c <0.70 and lower calibration). It was a retrospective analytical study, lasting one year, rom January-December 2017, in patients treated for tuberculosis in the department of pulmonology - phtisiology of the Ignace Deen teaching hospital. The discrimination model was determined by the area under the receiver operating characteristic curve (ROC) air under the curve(AUC). The correct calibration of the model was determined by an insignificant Hosmer-Lemeshow fit test.
Results: the inclusion cohort was made up of 1338 individuals, with an average age of 37.1 years ± 15.3 years and a sex ratio of 1.3 men for 1 woman. In our cohort, the diagnostic performance of the CABI score is moderate (AUC = 0.6885), but the calibration is not significant [H-L χ2 = 21.50 (p = 0.0059)]. On the other hand, with the new T for type of patient, A for age, B for body mass index and I for HIV infection (TABI) score, we obtain a good diagnostic performance (AUC = 0.75 95% CI [0.717 - 0.782]), a good calibration [HL χ2 = 12.0119 (p = 0.1507)], good specificity (60%) and good sensitivity (80%). The TABI score therefore has better effectiveness on Guinean patients but will however require external validation on another population.
Conclusion: this score can allow the early detection of tuberculosis patients at high risk of death, which can inform clinicians in their decision-making when these patients are started on treatment. However, further external validation studies will be required.
Tuberculosis is an infectious communicable disease caused by mycobacterium tuberculosis, it is the second leading cause of death following Human immunodeficiency virus (HIV) [1]. In 2018, the World Health Organization (WHO) lists an average of 10 million (9 to 11.100 million), new cases of tuberculosis with an estimated 1.5 million tuberculosis-related deaths worldwide, including 251.000 deaths in HIV positive patients. The average incidence rate is thus 130 cases per 100.000 inhabitants, of which nearly 87% of new cases are diagnosed in sub-Saharan Africa and South Asia. The disease recedes slowly each year with 58 million lives saved between 2000 and 2018 against 37 million between 2000 and 2013, thanks to effective treatment and diagnosis [2-4]. Unfortunately, in sub-Saharan Africa and more particularly in Guinea, and in the context of the HIV pandemic, mortality is constantly increasing [5]. Consequently, the identification of risk factors for death during anti-tuberculosis treatment becomes a priority for the various health systems which will be able to act on them, to improve or adapt patient management strategies. These risk factors are most often identified from multivariate prediction models defined as any combination of two or more factors (such as age, sex, stage of the disease, etc.) to estimate the probability of occurrence of a clinical event unfavorable for the patient, in this case death during anti-tuberculosis treatment.
Thus, in current practice, to simplify the identification of patients at high risk of death, these models are transformed into a risk score or prognostic score. Thus, very recently, a prognostic score for the risk of death during the anti-tuberculosis treatment called CABI (C for clinical forms of tuberculosis, A for age, B for body mass index and I for HIV infection) was developed in Cameroonian patients. The authors have shown that this score presents very good performances in terms of discrimination (c-index = 0.812 (CI95% = [0.784-0.841]) and calibration [HL χ2 = 6.44 (p = 0.60)] and a ratio of 4.8%. Although several other prognostic mortality scores linked to tuberculosis have been developed [6-11] and for some validated [6-11], the CABI score is one of the simplest and easily accessible in everyday practice. However, for this score to be used in current clinical practice as a patient monitoring tool, or to facilitate the selection and stratification of patients in future therapeutic trials, it needs to be validated on another independent sample. This process called external validation aims to assess the predictive capabilities of the CABI score on this new sample. In this work, we proposed to validate externally the CABI score in Guinean patients on anti-tuberculosis treatment. The objectives of this work were on the one hand, to validate the prognostic score (CABI) developed in Cameroon in Guinean patients treated for tuberculosis and on the other hand to improve the CABI score (substitution of one or more factors) only if its predictive capacities are not satisfactory (c-index <0.70 and lower calibration).
Material: our study focused on all patients admitted for pulmonary or extra-pulmonary tuberculosis treated in the pneumology department of the Ignace Deen teaching hospital and in the anti-tuberculosis treatment center of the quarry during our study period. Sources used for the study: Patient files, consultation registers, data collection sheet.
Study selection, participants, and outcome measure: it was a retrospective analytical study, lasting one year, rom January-December 2017, targeting all patients treated for pulmonary or extra-pulmonary tuberculosis during our study period. All records of patients aged 15 years and over and treated for pulmonary or extra-pulmonary tuberculosis according to WHO categories I or II were included, while records of patients under 15 years of age, multi-resistant tuberculosis (MRT) were excluded.
Data extraction: to extract the data, we carried out a documentary review on consultation registers and patient files, using a previously developed collection form.
Statistical analysis: the analysis of the data was carried out using statistical software “R”, V 3.6.1 (27/11/2019), (R Foundation for statistical computing, Vienna, Austria) and packages “rms "And" pROC. Data were summarized by size and frequency for categorical variables and by mean and standard deviation for quantitative variables. For the external validation of the CABI score, first the socio-demographic and clinical characteristics were compared between Guinean and Cameroonian patients, then a multivariate logistic regression model containing only the predictive factors of death from the CABI score was applied to our data. The performance of the model was evaluated by the c-index for discrimination and the graph between the predicted number of deaths by the model (on the abscissa) versus the observed number of deaths (on the ordinate) for the calibration. If, however the performance of the CABI score is not satisfactory on our data (c-index <0.70 and less good calibration with a significant Hosmer-Lemeshow test), then an improvement of this score was proposed either by adding or removing a or several factors of the model. This score improvement step will repeat all the steps for developing a prognostic score. A correction of the hyper optimism of the discriminative capacity via the c-index was estimated by the Bootstrap procedure with 1000 replications. A prognostic nomogram for this new score was derived, and an optimal threshold search using the methodology of the receiver-operating characteristics (ROC) curve via Youden's method [9] was applied. Finally, performance measures, including sensitivity, specificity, positive and negative predictive values (PPV and negative predictive value(NPV)), were then estimated for this optimal threshold.
Comparative characteristics of patients included in this study: comparatively to patients included in Cameroonian study (2250), the size of our inclusion cohort was 1338 patients. Table 1 summarize the comparative characteristics of patients included in this study.
General characteristics of patient included in this study: in our study, 197 deceases patients were found with an average age of 37.1 years ± 15.3 years including 754 men (56.4%) and 584 women (43.6%), i.e. a sex ratio of 1.3 men for 1 woman. The average BMI was 20.2 ± 4.1 kg/m2, the most represented clinical form was microscopic pulmonary tuberculosis 843 cases (64.2%), followed by extra pulmonary tuberculosis 237 cases (18.0 %) and at the end of negative tuberculosis pulmonary tuberculosis 234 cases (17.8%). The most represented registration group consisted of 1,205 new patients (90.0%), followed by 87 relapses (6.5%) and at the end of 46 failures (3.5%). Almost a third of our patients had HIV positive status (404 cases, 30.2%). Table 2 summarizes the characteristics of patients included in this study.
Determinant of death: univariate association of baseline characteristics with death in treatment are shown in Table 2. A total of 197 deaths (cumulative incidence 14.7%) was recorded during follow-up. Furthermore, 11.1% of deaths concerned new cases and 9.5% were smear positive pulmonary tuberculosis(PTB+). Age [OR 1.028 (95% CI: 1.018-1.037) per year higher], HIV infection [1.860 (1.360-2.538)], adjusted BMI [0.943 (0.906-0.981) per kg/m2 higher], failure patient type [OR 5.49 (95% CI: 2.96 - 10.06)] and relapse patient type [OR 3.57 (95% CI: 2.19 - 5.71)] were associated with mortality in univariate analysis; while gender ( p = 0.0622) and clinical form (p = 0.2150) were not. In mutually adjusted multivariable analysis, age, BMI, patient type, HIV infection (all p < 0.0001) remained significantly associated with mortality and were retained in the final model. Multivariate analysis of mortality factors for tuberculosis patients are surmised in Table 3.
Performance of the final model: we did not find no significant association between risk of death estimated by the final model and the observed rates of death, across the continuum of predicted probability (Figure 1). The Hosmer and Lemeshow χ2 was 21.50 and the accompanying p-value was 0.0059. The discrimination of the model in the derivation sample (apparent discrimination) was not good with a c-statistic of 0.688 (95% CI: 0.65-0.73).
Determinant of death for TABI model: since we found a relationship with type of patient, we included it in a mutually adjusted multivariable analysis in place of clinical form. We fund that, Age, BMI, patient type and HIV infection (all p < 0.0001) remained significantly associated with mortality and were retained in our final TABI model. Multivariate analysis of mortality factors for tuberculosis patients are surmised in Table 4.
Performance on the final model TABI: we find a significant association between risk of death estimated by the final model TABI and the observed rates of death, across the continuum of predicted probability (Figure 2). The Hosmer and Lemeshow χ2 was 12.0119 and the accompanying p-value was 0.1507. The discrimination of the model in the derivation sample (apparent discrimination) was good with a c-statistic of 0.75 (95% CI: 0.717-0.782) as shown in Figure 3.
Point scoring system: the nomogram showing the point scores corresponding to levels of each predictor in the final model is shown in Figure 4. The ranged of point scores was 10-90 for age, 10- 45 for BMI, 0-28 for HIV infection and 0-69 for type of patient. The total points ranged from 67 to 244. The optimal point-score for predicting death was 57. Corresponding performance measures were 80% (sensitivity), 60% (specificity). The performance of the point-scoring system at different thresholds is summarized in Table 5.
Evaluation of the risk of death with TABI model: based on the optimal point-score of 57 in the derivation sample, the tree levels of risk described in Table 6 were established. Group I correspond to low risk and Group III to critical risk. As expected, death rate increased across Group I, II, and III. Elements of interpretation are summarizing in the same table.
Ethics approval and consent to participate: we confirm that the approval of an institutional review board was not required for this work and we have read the Journal's position on issues involved in ethical publication and affirm that this work is consistent with those guidelines.
In this study, we tried to validated (externally) and then improved the CABI prognostic score for tuberculosis mortality in patients on treatment using 4 demographic and clinical characteristics (age, weight, clinical form of TB, and HIV status), which are available in current practice in any healthcare establishment. The characteristics of patients included in our study were close to those obtained in the development cohort by Pefura-Yone et al. in 2017, which had found predominance´s identical to that of our inclusion cohort except for proportion of microscopically negative tuberculosis in the two populations. Univariate analysis in our cohort confirmed the variables Age, BMI, Type of patient, and HIV serology as associated with mortality (p-value <5%) but not Clinical form as it was in the Cameroonian model. Our results are a bite different with those found in previous studies which had already identified these factors as being associated with mortality in patients treated for tuberculosis including clinical form [8,12-23]. Overall, in our cohort, the predictive capacities of the CABI score were not satisfactory with moderate discrimination (AUC = 0.688 (CI95% = [0.65; 0.73], and poor calibration [HL χ2 = 21.50 (p = 0.0059)]. The CABI score therefore has moderate efficacy on Guinean patients and probably requires recalibration. Our results are different from those obtained in Cameroon by Pefura-Yone et al. in 2017, which had obtained good performance diagnosis (AUC = 0.808 (95% CI = [0.784-0.841])) and a good calibration [HL χ2 = 6.44 (p = 0.60)]. This can be explained by two reason; first, the number of variables included in the final model were less than those included in Cameroon (tree vs four). Secondly, although most of the characteristics were comparable in the two populations, however, there is a difference in the proportion of microscopically negative tuberculosis in the two populations (9.3% vs. 17.8%). In view of this moderate result, we tried to improve the CABI score by developing another score by replacing the clinical form (PTB+, smear positive pulmonary tuberculosis (PTB-), extra-pulmonary tuberculosis (ETB)), by the type of patient (New case, Failure, Relapse) to obtain the "TABI" score (T = type of patient, A = age, B = Body mass index and I = HIV infection), since no association with dead was fund with clinical form in our study.
We validated our TABI score internally since it has very good internal validity. Air under the curve (AUC) is a good measure to assess the overall performance of a clinical score. In general, an AUC> 0.7 is considered clinically useful [8]. This score can help early identify patients at high risk of death, which could improve their chance of recovery. However, decisions about how to use the score and what thresholds to apply for decision-making require careful consideration as it depends on the context and is largely determined by the clinical characteristics of the patients. To interpret the TABI score, our patients were classified into three categories: low, medium, and high. Thus, patients with a total score below 57 points will have a low risk, as long as patients with a score greater than or equal to 57, but less than 100 will have a medium risk and when the score is equal to or greater than 100 the risk of death during treatment is high. However, our study has certain limitations; First, the source of our data, which was collected retrospectively, and then, the use of univariate analysis as a method for selecting the variables that we included in our model. On the other hand, the TABI score was developed and validated internally on our sample, it will be necessary to validate it on another independent sample. Despite the limitations observed, several strong points make the TABI prognostic score distinct from the other scores developed to date. In particular, the dataset from which it was developed, which is representative of its population, the use of socio-demographic characteristics and clinical parameters systematically collected by most tuberculosis treatment centers from the first visits. This TABI score can be used to identify patients with a high risk of mortality. Finally, our TABI score presents good predictive abilities and provides a simple reading grid to help the clinician in his decision-making. Other prognostic scores for tuberculosis have already been developed and some have been validated externally [8,13,14].
At the end of our study, the objective of which was to validate the CABI prognostic score, it emerges that this score gives moderate results when it is applied to a cohort of Guinean patients. Thus, we do not recommend its use in current practice in tuberculosis patients in Guinea. To go further, we proposed to develop a new prognostic score called TABI which presents better performances.
What is known about this topic
- A prognostic score for the risk of death during the anti-tuberculosis treatment called CABI (C for clinical forms of tuberculosis, A for age, B for body mass index and I for HIV infection) was developed in Cameroonian patients and the authors have shown that this score presents very good performances in terms of discrimination and calibration;
- However, for this score to be used in current clinical practice as a patient monitoring tool, or to facilitate the selection and stratification of patients in future therapeutic trials, it needs to be validated on another independent sample. This process called external validation aims to assess the predictive capabilities of the CABI score on this new sample.
What this study adds
- We found a relationship with type of patient then, we included it in a mutually adjusted multivariable analysis in place of clinical form. We fund that, Age, BMI, patient type and HIV infection remained significantly associated with mortality, so we retained them in our final TABI model (T = type of patient, A = age, B = Body mass index and I = HIV infection);
- Our TABI score can allow the early detection of tuberculosis patients at high risk of death, which can inform clinicians in their decision-making when these patients are started on treatment.
The authors declare no competing interests.
All authors have read and approved the manuscript. Gerard Christian Kuotu, Boubacar Djelo Diallo and Alhassane Diallo had the idea for the article and performed the literature search, Alhassane Diallo performed the statistical analysis, Gerard Christian Kuotu, Boubacar Djelo Diallo, Alhassane Diallo, Demba Toure, drafted the article, and, Lansana Mady Camara critically revised the work. All the authors have read and agreed to the final manuscript.
We thank the National Tuberculosis Control Program for its collaboration. We thank Dr. Moukam Lower Bride Barth (Faculty of Health Sciences and Techniques, Gamal Abdel Nasser University in Conakry, Pneumo-Phthisiology Department, Conakry University Hospital, Ignace Deen National Hospital in Conakry, Guinea) for help in revising the English of the manuscript.
Table 1: comparative characteristics of patients included in this study
Table 2: univariate logistic regression of mortality factors for tuberculosis patients
Table 3: multivariate analysis of mortality factors for tuberculosis patients (CABI model)
Table 4: multivariate analysis of mortality factors for tuberculosis patients (TABI model)
Table 5: elements of prognosis reading of the TABI score
Table 6: classification of risk groups with the TABI score
Figure 1: calibration figures of tuberculosis prognostic score with the CABI model
Figure 2: calibration figures of tuberculosis prognostic score with the TABI model
Figure 3: ROC curve for predicting the final model (TABI model)
Figure 4: nomogram (TABI model)
- Mjid M, Cherif J, Ben Salah N, Toujani S, Ouahchi Y, Zakhama H et al. Epidemiology of tuberculosis. Rev Pneumol Clin. 2015;71(2-3):67-72. PubMed | Google Scholar
- Saiman L, San Gabriel P, Schulte J, Vargas MP, Kenyon T, OnoratoI. Risk factors for latent tuberculosis infection among children in New York City. Pediatrics. 2001 May;107(5):999-1003. PubMed | Google Scholar
- WHO. Global tuberculosis report 2019. 2019.
- World Health Organization. World health statistics 2014 Geneva, Switzerland. 2014.
- United Nations. The Millennium Development Goals report 2010. New York, NY, USA: UN. 2010.
- Pefura-Yone EW, Balkissou AD, Mangoh L, Touyem-Lonla J, Kengne AP. Validation externe du score pronostic de la tuberculose CABI dans la région de l´Ouest-Cameroun. Rev Mal Respir. 2018;35:A40. Google Scholar
- Collett D. Modelling binary data. 2nd ed. New York: Chapman & Hall/CRC. 2003. Google Scholar
- Hosmer D, Lemeshow S. Applied logistic regression. 2nd ed. New York: Willey. 2000. Google Scholar
- Youden WJ. Index for rating diagnostic tests. Cancer. 1950 Jan;3(1):32-5. PubMed | Google Scholar
- Gentilini M, Caumes E, Danis M, Mouchet J, Duflo B, Lagardere B et al. Tuberculose. Médecine Tropicale. 2001;(5):309-324.
- Tripepi G, Jager KJ, Dekker FW, Zoccali C. Diagnostic methods 2: receiver operating characteristic (ROC) curves. Kidney Int. 2009;76(3):252-6. PubMed | Google Scholar
- Justice AC, Covinsky KE, Berlin JA. Assessing the generalizability of prognostic information. Ann Intern Med. 1999 Mar 16;130(6):515-24. PubMed | Google Scholar
- Abongomera C, Ritmeijer K, Vogt F, Buyze J, Mekonnen Z, Admassu H et al. Development and external validation of a clinical prognostic score for death in visceral leishmaniasis patients in a high HIV co-infection burden area in Ethiopia. PloS One. 2017;12(6):e0178996. PubMed | Google Scholar
- Bastos HN, Osório NS, Castro AG, Ramos A, Carvalho T, Meira L et al. A prediction rule to stratify mortality risk of patients with pulmonary tuberculosis. PloS One. 2016;11(9):e0162797. PubMed | Google Scholar
- Nguyen DT, Jenkins HE, Graviss EA. Prognostic score to predict mortality during TB treatment in TB/HIV co-infected patients. PloS One. 2018;13(4):e0196022. PubMed | Google Scholar
- Gupta-Wright A, Corbett EL, Wilson D, van Oosterhout JJ, Dheda K, Huerga H et al. Risk score for predicting mortality including urine lipoarabinomannan detection in hospital in patients with HIV-associated tuberculosis in sub-Saharan Africa: derivation and external validation cohort study. PLoS Med. 2019;16(4):e1002776. PubMed | Google Scholar
- Penn-Nicholson A, Hraha T, Thompson EG, Sterling D, Mbandi SK, Wall KM et al. Discovery and validation of a prognostic proteomic signature for tuberculosis progression: a prospective cohort study. PLoS Med. 2019;16(4):e1002781. PubMed | Google Scholar
- Bright TJ, Wong A, Dhurjati R, Bristow E, Bastian L, Coeytaux RR et al. Effect of clinical decision-support systems: a systematic review. Ann Intern Med. 2012;157(1):29-43. PubMed | Google Scholar
- Nguyen DT, Graviss EA. Development and validation of a prognostic score to predict tuberculosis mortality. J Infect. 2018;77(4):283-90. PubMed | Google Scholar
- Horita N, Miyazawa N, Yoshiyama T, Sato T, Yamamoto M, Tomaru K et al. Development and validation of a tuberculosis prognostic score for smear-positive in-patients in Japan. Int J Tuberc Lung Dis Off J Int Union Tuberc Lung Dis. 2013;17(1):54-60. PubMed | Google Scholar
- Kim DK, Kim HJ, Kwon SY, Yoon HI, Lee CT, Kim YW et al. Nutritional deficit as a negative prognostic factor in patients with miliary tuberculosis. Eur Respir J. oct 2008;32(4):1031-6. PubMed | Google Scholar
- Keane VP, de Klerk N, Krieng T, Hammond G, Musk AW. Risk factors for the development of non-response to first-line treatment for tuberculosis in southern Vietnam. Int J Epidemiol. 1997;26(5):1115-20. PubMed | Google Scholar
- Gupta-Wright A, Corbett EL, Wilson D, van Oosterhout JJ, Dheda K, Huerga H et al. Risk score for predicting mortality including urine lipoarabinomannan detection in hospital in patients with HIV-associated tuberculosis in sub-Saharan Africa: Derivation and external validation cohort study. PLoS Med. 2019;16(4):e1002776. PubMed | Google Scholar
Search
This article authors
On Pubmed
On Google Scholar
Citation [Download]
Navigate this article
Similar articles in
Warning: file_get_contents(): SSL operation failed with code 1. OpenSSL Error messages: error:0A000126:SSL routines::unexpected eof while reading in /home/panafric/panafrican-med-journal.com/content/article/index_article_common.php on line 546
Warning: file_get_contents(): SSL: Success in /home/panafric/panafrican-med-journal.com/content/article/index_article_common.php on line 546