Use of HBA1c and potentiality of gender, missed medication and fasting glucose in the prediction of poor glycemic control in resource-limited setting; a clinic-based case-control study
Daniel Wilfred Gunda, Hussein Abbas Bandali, Evangelista Kenan Malindisa, Benson Richard Kidenya
Corresponding author: Daniel Wilfred Gunda, Department of Internal Medicine, Catholic University of Health and Allied Sciences, 1464 Mwanza, Tanzania
Received: 02 Apr 2020 - Accepted: 26 Jul 2020 - Published: 14 Aug 2020
Domain: Endocrinology
Keywords: HBA1c, fasting hyperglycemia, diabetes Mellitus type 2, uncontrolled diabetes, northwestern Tanzania, resource limited setting
©Daniel Wilfred Gunda et al. PAMJ-One Health (ISSN: 2707-2800). This is an Open Access article distributed under the terms of the Creative Commons Attribution International 4.0 License (https://creativecommons.org/licenses/by/4.0/), which permits unrestricted use, distribution, and reproduction in any medium, provided the original work is properly cited.
Cite this article: Daniel Wilfred Gunda et al. Use of HBA1c and potentiality of gender, missed medication and fasting glucose in the prediction of poor glycemic control in resource-limited setting; a clinic-based case-control study. PAMJ-One Health. 2020;2:22. [doi: 10.11604/pamj-oh.2020.2.22.22624]
Available online at: https://www.one-health.panafrican-med-journal.com/content/article/2/22/full
Research 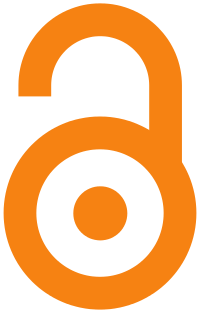
Use of HBA1c and potentiality of gender, missed medication and fasting glucose in the prediction of poor glycemic control in resource-limited setting; a clinic-based case-control study
Use of HBA1c and potentiality of gender, missed medication and fasting glucose in the prediction of poor glycemic control in resource-limited setting; a clinic-based case-control study
Daniel Wilfred Gunda1,2,&, Hussein Abbas Bandali1, Evangelista Kenan Malindisa3, Benson Richard Kidenya4
&Corresponding author
Introduction: diabetes mellitus (DM) type 2 is a rapidly growing health problem in the world especially in resource limited countries. Even with availability of affordable medication still a large proportion of patients don´t attain a well-controlled glycemic state thus putting them at high risk of morbidity and mortality due to irreversible complications. Availability of glycated hemoglobin test (HBA1c) for better monitoring of glycemic control is still a setback in most resource restricted setting including Tanzania. This study was designed to determine the magnitude of uncontrolled DM type 2 using HBA1c and assess the utility of different factors in sorting patients at high risk of having uncontrolled DM in a clinic based setting in north western Tanzania.
Methods: this was a case control study involving diabetes type 2 patients at Bugando Medical Centre. A cross sectional measurement of glycated hemoglobin (HBA1c) and fasting glucose were done, medical history and adherence status to anti diabetes were assessed. Data analysis was done using STATA 13. Uncontrolled Diabetes was defined as an HBA1c of more than 7.0 and its correlates were assessed by logistic regression model. The predictive ability of independent variables was determined by calculating their sensitivity and specificity.
Results: in total 229 patients were included in this study, where 114 (49.8%; 95%CI: 43.3-56.3) had uncontrolled DM2 by HBA1c. In this study uncontrolled DM was independently associated with female gender, (AOR: 2.1; 95%CI: 1.1-3.9; p=0.022), frequently missed medications (AOR: 1.1; 95%CI: 1.03-1.2; p=0.006), and higher fasting median blood glucose, (10.5 vs. 6.9; AOR: 7.3; IQR: 3.7-14.7; P<0.001). Fasting glucose had higher predictive values to uncontrolled DM2 (sensitivity: 79.0%; 95%CI: 70.3-86.0; specificity: 84.4%; 95%CI: 76.4-90.4, cut point: 8.5; area under ROC curve: 0.8584)as compared to gender and missed medications.
Conclusion: uncontrolled DM type 2 is common in Notherwestern Tanzania, and is well predicted by fasting hyperglycemia. Fasting hyperglycemia can be used in selecting patients that could benefit from timely intensification of treatment where HBA1c is not available.
Diabetes mellitus (DM) is a rapidly growing global health problem which carries high morbidity and mortality, especially in low-income countries including Africa. In 2014 about 442 million adults had DM with a global prevalence of 8.5% as compared to 108million (4.7%) in 1980 [1]. By 2030, it is estimated that more than 400 million (85.0%) of DM patients will be living in developing countries [2,3]. In Sub Saharan Africa DM is about 12% of the general population where diabetes mellitus type two accounts for more than 90% of all diabetic cases [4]. In Tanzania, between 4.3-5.3% of the general population was estimated to have DM in 2016 [5,6]. The mortality due to DM is unacceptably high. For instance, in 2012 alone over 3.7 million people died due to DM and nearly a half, (43%) of these deaths occurred before the age of 70 mainly due to failure to achieve optimal glycemic control [7]. Uncontrolled DM increases the risk of recurrent infection which may significantly contribute to morbidity and mortality [8-10]. Several other irreversible diabetic mellitus related complications also contribute to high morbidity and mortality including coronary arterial diseases, cerebral vascular accident, chronic renal failure, diabetic foot diseases with leg amputation, vision loss and neuropathy among others [11,12]. Studies among patients with Diabetes type 2 indicate that poorly controlled DM is a very frequent problem where some studies have reported a prevalence of up to 86% of their participants [13-15]. The risk of uncontrolled DM is highest among young patients, those who are un-compliant to medications, those with longer diabetic periods, and among those with longer travel distances to the health facility among others [16-24]. The current study describes the magnitude and potential discriminators of uncontrolled DM using HBA1c among adult DM2 patients at Bugando. We believe this data is important in devising potential strategies to achieve overall optimization of care of patients with DM 2 in diabetic care settings similar to ours.
This was a hospital-based case-control study, which was done between September 2018 and July 2019 as an elective research activity. The study was conducted at Bugando Medical Centre (BMC) in the northwestern part of Tanzania. The diabetes clinic exists as an integral part of internal medicine. Diabetic patients diagnosed within and those referred in from catchment hospitals are routinely monitored in this clinic on a monthly base. Patients who are found to have uncontrolled sugars, the potential triggers of uncontrolled diabetes are usually sought including un-compliance to medications and infections with subsequent adjustment of anti-diabetes doses. All adult diabetic type 2 patients on treatment were involved in this study. A minimum sample size of 185 was estimated from Kish and Lisle formula assuming 86% of the study participants had uncontrolled DM as found previously [25] with an allowable error of 0.05 at 95% Confidence Interval (CI). Patients were invited to participate in the study and the informed consent was obtained. Information regarding demographic data, type of medications and doses, missed medications, clinic attendance status and duration of DM were recorded and then all patients had a test for fasting blood glucose (FBG) and glycated adult hemoglobin (HBA1c).
Data were computerized using Epi data version 3.1 and STATA version 13 (Stata Corp LP, college station, TX) was used for data analysis. Continuous variables were expressed as medians with interquartile range (IQR) while categorical variables were expressed as proportions with percentages. Uncontrolled DM was defined as an HBA1c level of more than 7% as described previously [26] and was expressed as a percentage with 95% Confidence Interval(CI). The odds ratio (OR) with 95%CI of potential explanatory factors were calculated using univariate followed by multivariate logistic regression to assess the extent of association between different variables and the outcome of interest. In the first model, the factors were considered for inclusion into the final model if p< 0.2. The level of independent association was set at p<0.05 in the final model and the goodness of fit of the final logistic model was subsequently assessed. Based on previous knowledge and our own experience, Sociodemographic information, medical compliance, medication type, and fasting glucose were included in the logistic model [16-24]. The sensitivity and specificity of the final explanatory factors were determined to assess its utility in predicting uncontrolled DM in this subgroup of patients. To obtain the best cut point for continuous variables, a Receiver operating characteristic (ROC) curve was used.
Ethical clearance: the permission to conduct and publish the results from this research was sought from the Catholic University of Health and Allied Sciences (CUHAS)/ Bugando Medical Center joint ethical committee. Patients who didn´t consent were not reprimanded of their access to DM care. The patient´s files were handled by the researchers alone and the patients´ identifiers including names and registration numbers were not included in the final analysis to further maintain confidentiality.
General study characteristics: a total of 229 patients were enrolled in this study. The majority, 125 (54.6%) were female participants with a median age of 59 (IQR: 53-67) years. The median duration of DM2 was 2 (IQR: 2-4) years with a median fasting glucose of 8 (IQR: 6.7-10.7) mmol/dL. About a third, 143(62.5%) of participants had fasting glucose higher than 7.1mmol/dL on the day of enrolment with a median number of missed drugs of 2 (IQR: 0-6) days (Table 1).
Prevalence and associated factors of uncontrolled DM2 among 229 participants: in the current study, 114 (49.8%; 95%CI: 43.3-56.3) participants met HBA1c criteria for uncontrolled diabetes (Table 1). The odds of having uncontrolled DM by HBA1c were independently increased among female patients, (AOR: 2.1; 95%CI: 1.1-3.9; p=0.022), frequently missed medications (AOR: 1.1; 95%CI: 1.03-1.2; p=0.006), and with higher median fasting blood glucose, (10.5 vs. 6.9; AOR: 7.3; IQR: 3.7-14.7; P<0.001). The difference in the distribution of other factors was not different statistically (Table 2) and the assessment of the goodness of fit of the final model demonstrated no gross lack of fitness (Hosmer-Lemeshow chi2 (8): 8.31; Prob > chi2: 0.4041, area under ROC curve: 0.8086) (Figure 1). The assessment of the prediction ability indicated that elevated fasting blood glucose of more than 8.5mmol/dL had the highest sensitivity (79.0%; 95%CI: 70.3-86.0) and specificity (84.4%; 95%CI: 76.4-90.4) with an area under ROC curve of 0.8584 as compared to female gender and missed medications (Table 3).
The objective of this study was to determine the prevalence and associated factors of uncontrolled DM type 2 by using HBA1c levels and assess the predictive ability of the independent factors. In this study, 114 (49.8%) of the studied participants were found to have uncontrolled DM which was more likely to occur among female participants, with frequently missed medications and higher median fasting blood glucose. Median fasting glucose of more than 8.5 demonstrated both high sensitivity and specificity in predicting uncontrolled DM 2 as determined by HBA1c. The prevalence of uncontrolled DM2 in this study is similar to the prevalence of 43.0% from the USA [24] and 55.3% from Trinidad [27]. However, our finding is higher than a prevalence of 16.7% reported from Israel [28] and 38.9% reported from Pakistan [14]. Higher prevalence of uncontrolled DM2 was reported in Hawaii, (68.5% vs. 49.8%) [16], urban part of Dar es salaam (69.7% vs. 49.8%) [20], Ethiopia, (80.0% vs. 49.8%) [22] and Ghana (86.0% vs. 49.8%) [25]. Even with these differences in the prevalence, these findings suggest that uncontrolled DM2 is a wide spread problem which might be even higher among resource-limited countries. Prior studies had suggested that persistent hyperglycemia among diabetic patients increases the independent risk of both micro and macro vascular complications [29]. The findings in the current study suggests that about 50.0% of our participants had persistent hyperglycemia for over 3 months, putting them at highest risk of morbidity due irreversible chronic diabetic complications [30]. Thus deliberate planning of immediate intensive glycemic control in this subgroup of patients is important in scaling down the unfavorable outcome following poor glycemic control [31].
To identify patients at risk of poor glycemic control several factors were assessed for their independent association with uncontrolled diabetes mellitus. Several other previous studies have reported similar findings that female gender is associated with poor glycemic control including reports by Nuez et al. from USA [32] and Apparico et al. from Trinidad [27]. Similar to our current study missing drugs were also significantly associated with uncontrolled DM in a study by Tubiana-Rufi et al. in France[18], in Ethiopia [19] and urban Dar es salaam [20]. In agreement with our study also, a positive correlation between higher fasting blood glucose and higher glycated HBA has been reported in several other studies among adult patients with diabetes mellitus type 2 [33-35]. Since HBA1c measurements are not routinely done in our setting similar to most resource-limited settings [36], a simple, and rapid practically cheap tool is important in facilitating isolation of patients at high risk of having uncontrolled DM type 2. In our study fasting blood sugar was found to have highest sensitivity and specificity in predicting persistent hyperglycemia by HBA1c. Similar findings were reported in another study where it was found that persistent fasting hyperglycemia also predicts better uncontrolled diabetes (area under ROC curve: 0. 92) [37] suggesting that among those on medications, fasting hyperglycemia could potentially discriminate patients with uncontrolled diabetes mellitus. With a linear correlation between fasting hyperglycemia and HBA1c among DM type 2 patients, Al-Lawati et al. indicated that fasting hyperglycemia had a sensitivity and specificity of up to 70.7% and 82.7% respectively in predicting uncontrolled DM type 2 (AUROC curve:0.80; 95%CI:0.79-82) similar to our study [38]. These findings suggest that in setting where HBA1c is not readily obtainable for routine use fasting blood sugars levels may be used to identify patients who are likely to have uncontrolled type 2 diabetes and plan on strengthening of treatment to mitigate morbidity from long- term DM complications.
The current study is liable to some limitations; including the fact that this is a single-center study, its results may not be generalizable. Since this was a cross sectional study patients follow up was not done. However, even with these limitations, the findings from this study are still important, especially in resource-limited settings where HBA1c is not readily done. The current results suggest that fasting hyperglycemia is potentially useful in selecting patients at high risk of having uncontrolled DM2 and thus can be planed for intensified glycemic control. Longitudinal studies to further assess the performance of fasting glucose with larger sample sizes are warranted.
What is known about this topic
- Diabetes mellitus type 2 in a rapidly growing problem in resource limited setting;
- Poor glycemic control dramatically increases the morbidity and mortality in DM 2 patients;
- HBA1c is feasibly used in resource rich countries for long term of monitoring of glycemic control.
What this study adds
- Uncontrolled DM 2 is a common encounter in resource limited countries as well;
- Fasting blood glucose can reliably select patients with poor long term glycemic control who would benefit from immediate initiation intensified glycemic in setting where HBA1c is not readily obtainable.
The authors declare no competing interests.
DWG&EKM: participated in designing of the study; HAB; acquired the data; DWG& BRK: did data analysis and interpretation; DWG: did manuscript drafting. All the authors significantly reviewed the manuscript for its intellectual content and agreed on the final version.
The authors would like to acknowledge the great support given by the department of medical records and the diabetes team at BMC during the enrolment and retrieval of patients´ information.
Table 1: general study characteristics among 229 DM type 2 participants
Table 2: associated factors of uncontrolled DM 2 among 229 study participants
Table 3: prediction of uncontrolled DM by gender, missed drug and fasting glucose
Figure 1: assessment of the goodness of fit for the final logistic model
- Roglic Gojka. WHO Global report on diabetes: a summary. International Journal of Noncommunicable Diseases. 2016;1(1):3. Google Scholar
- Wild H Sara, Roglic G, Anders G, Richard S, Hilary K. Global prevalence of diabetes: estimates for the year 2000 and projections for 2030. Diabetes Care. 2004;27(5):1047-53. PubMed | Google Scholar
- Shaw JE, Sicree RA, Zimmet PZ. Global estimates of the prevalence of diabetes for 2010 and 2030. Diabetes Res Clin Pract. 2010;87(1):4-14. PubMed | Google Scholar
- Hall Victoria, Reimar WT, Ole H, Nicholai L. Diabetes in Sub Saharan Africa 1999-2011: epidemiology and public health implications. A systematic review. BMC Public Health. 2011 Jul 14;11:564. PubMed | Google Scholar
- WHO. Diabetes country profiles 2016. WHO. 2016.
- Stanifer JW, Charles RC, Gerald JM, joseph RE, Venance M, Honest M et al. Prevalence, Risk Factors, and Complications of Diabetes in the Kilimanjaro Region: A Population-Based Study from Tanzania. PLoS One. 2016;11(10):e0164428. PubMed | Google Scholar
- WHO. WHO global report on diabetic melitus. WHO. 2016.
- Shah BR, Hux JE, Quantifying the risk of infectious diseases for people with diabetes. Diabetes Care. 2003; 26(2): 510-3. Google Scholar
- Kornum JB, Reimar WT, Anders R, Hans-Henrik L, Henrik CS, Henrik TS. Diabetes, glycemic control, and risk of hospitalization with pneumonia: a population-based case-control study. Diabetes Care. 2008;31(8):1541-5. PubMed | Google Scholar
- Jeon CY, Murray MB. Diabetes mellitus increases the risk of active tuberculosis: a systematic review of 13 observational studies. PLoS Med. 2008; 5(7):e152. Google Scholar
- Mattila TK, de Boer A. Influence of intensive versus conventional glucose control on microvascular and macrovascular complications in type 1 and 2 diabetes mellitus. Drugs. 2010;70(17):2229-45. PubMed | Google Scholar
- Chawla A, Chawla R, Jaggi S. Microvasular and macrovascular complications in diabetes mellitus: Distinct or continuum. Indian J Endocrinol Metab. 2016;20(4):546-51. PubMed | Google Scholar
- Zgibor JC, Gieraltowski LB, Talbott EO, Fabio A, Sharma RK, Hassan K. The association between driving distance and glycemic control in rural areas. J Diabetes Sci Technol. 2011;5(3):494-500. PubMed | Google Scholar
- Siddiqui FJ, Avan BI, Mahmud S, Nanan DJ, Jabbar A, Assam PN. Uncontrolled diabetes mellitus: prevalence and risk factors among people with type 2 diabetes mellitus in an Urban District of Karachi, Pakistan. Diabetes Res Clin Pract. 2015 Jan;107(1):148-56. PubMed | Google Scholar
- Fiagbe J, Bosoka S, Opong J, Takramah W, Axame WK, Owusu R et al. Prevalence of controlled and uncontrolled diabetes mellitus and associated factors of controlled diabetes among diabetic adults in the hohoe municipality of Ghana. Diabetes Management. 2017;7(5). Google Scholar
- Juarez DT, Sentell T, Tokumaru S, Goo R, Davis JW, Mau MM. Factors associated with poor glycemic control or wide glycemic variability among diabetes patients in Hawaii, 2006-2009. Prev Chronic Dis, 2012;9:120065.
- Koro CE, Bowlin SJ, Bourgeois N, Fedder DO. Glycemic control from 1988 to 2000 among U.S. adults diagnosed with type 2 diabetes: a preliminary report. Diabetes Care, 2004;27(1):17-20. PubMed | Google Scholar
- Tubiana-Rufi N, Leila M, Paul C, Judith C. Risk factors for poor glycemic control in diabetic children in France. Diabetes Care. 1995;18(11):1479-82. PubMed | Google Scholar
- Kassahun T, Eshetie T, Gesesew H. Factors associated with glycemic control among adult patients with type 2 diabetes mellitus: a cross-sectional survey in Ethiopia. BMC Res Notes. 2016;9:78. PubMed | Google Scholar
- Kamuhabwa AR, Charles E. Predictors of poor glycemic control in type 2 diabetic patients attending public hospitals in Dar es Salaam. Drug Healthc Patient Saf. 2014;6:155-65. PubMed | Google Scholar
- Sayed MH, Hegazi MA, Khairyah A, Khairya M, El-Deek BS, Hala G et al. Risk factors and predictors of uncontrolled hyperglycemia and diabetic ketoacidosis in children and adolescents with type 1 diabetes mellitus in Jeddah, western Saudi Arabia. J Diabetes. 2017; 9(2):190-199. PubMed | Google Scholar
- Tekalegn Y, Tedla K, Wondimu A. Magnitude of glycemic control and its associated factors among patients with type 2 diabetes at Tikur Anbessa Specialized Hospital, Addis Ababa, Ethiopia. PLoS One, 2018;13(3): e0193442. Google Scholar
- Alzaheb RA, Altemani AH. The prevalence and determinants of poor glycemic control among adults with type 2 diabetes mellitus in Saudi Arabia. Diabetes Metab Syndr Obes. 2018;11:15-21. PubMed | Google Scholar
- Strauss K, Charles M, Austin T, Benjamin L. Driving distance as a barrier to glycemic control in diabetes. J Gen Intern Med. 2006;21(4):378-80. PubMed | Google Scholar
- Fiagbe J, Bosoka S, Opong J, Takramah W, Axame WK, Owusu R et al. Prevalence of controlled and uncontrolled diabetes mellitus and associated factors of controlled diabetes among diabetic adults in the Hohoe municipality of Ghana. Diabetes Management. 2017;7(5):343-354. Google Scholar
- Yusuf K, Aysegul I, Nuket BK, Nurcan U, Ganime C, Iskender E et al. Relationship between the poor glycemic control and risk factors, life style and complications. Biomedical Research. 2017;28(4). PubMed | Google Scholar
- Apparico, N, Natalie C, Giana H, JasonS, Roger S, SimoneW et al. How well controlled are our type 2 diabetic patients in 2002: an observational study in North and Central Trinidad. Diabetes Res Clin Pract. 2007;75(3):301-5. PubMed | Google Scholar
- Feldman BS, Chandra JC, Morton L, Moshe BH, Shepherd RS, Haim B et al. Defining the role of medication adherence in poor glycemic control among a general adult population with diabetes. PLoS One. 2014 Sep 26;9(9):e108145. PubMed | Google Scholar
- Shogbon AO, Levy SB. Intensive glucose control in the management of diabetes mellitus and inpatient hyperglycemia. Am J Health Syst Pharm. 2010;67(10):798-805. PubMed | Google Scholar
- Rohlfing, CL, Rohlfing, Hsiao-Mei W, Randie RL, Jack DE, Alethea T et al. Defining the relationship between plasma glucose and HbA1c: analysis of glucose profiles and HbA1c in the Diabetes Control and Complications Trial. Diabetes Care. 2002;25(2):275-278. PubMed | Google Scholar
- Fullerton B, Jeitler K, Seitz M, Horvath K, Bergholt A, SiebenhoferA. Intensive glucose control versus conventional glucose control for type 1 diabetes mellitus. Cochrane Database Syst Rev. 2014 Feb 14;2014(2):CD009122. PubMed | Google Scholar
- Nunez MA, Yarandi H, Nunez-Smith M, Self-management among patients living with diabetes in the United States Virgin Islands. J Health Care Poor Underserved, 2011; 22(1):271-83. PubMed
- Stamouli M, Abraham P, Antonia M, Anastasios S, Ioanaa P, Evangelia M et al. Identifying the Relation between Fasting Blood Glucose and Glycosylated Haemoglobin Levels in Greek Diabetic Patients. Peertechz J Cytol Pathol. 2016;33(025):10-12. Google Scholar
- Arthur FK, Yeboah FA, Nsiah K, Nkrumah PK, Afreh KA, Agyenim-Boateng K. Fasting blood glucose and glycosylated haemoglobin levels in randomly selected Ghanaian diabetic patients-the clinical implications. Journal of Science and Technology (Ghana). 2005;25(2):13-17. PubMed | Google Scholar
- Sheikhpour R, Sadeghian F, Rajabi S. Correlation between glycated hemoglobin, serum glucose and serum lipid levels in type 2 diabetes. Iranian Journal of Diabetes and Obesity. 2013;5(1):12-15. Google Scholar
- Park PH, Pastakia SD. Access to Hemoglobin A1c in Rural Africa: a Difficult Reality with Severe Consequences. J Diabetes Res. 2018;2018:6093595. PubMed | Google Scholar
- Inoue K, Inoue M, Matsumoto M, Akimoto K. Persistent fasting hyperglycaemia is more predictive of Type 2 diabetes than transient fasting hyperglycaemia. Diabetic Medicine. 2012;29(7):e75-e81. PubMed | Google Scholar
- Al-Lawati JA, Al-Lawati AM. The utility of fasting plasma glucose in predicting glycosylated hemoglobin in type 2 diabetes. Annals of Saudi medicine. 2007;27(5):347-351. PubMed | Google Scholar
Search
This article authors
On Pubmed
On Google Scholar
Citation [Download]
Navigate this article
Similar articles in
Warning: file_get_contents(): SSL operation failed with code 1. OpenSSL Error messages: error:0A000126:SSL routines::unexpected eof while reading in /home/panafric/panafrican-med-journal.com/content/article/index_article_common.php on line 546
Warning: file_get_contents(): SSL: Success in /home/panafric/panafrican-med-journal.com/content/article/index_article_common.php on line 546